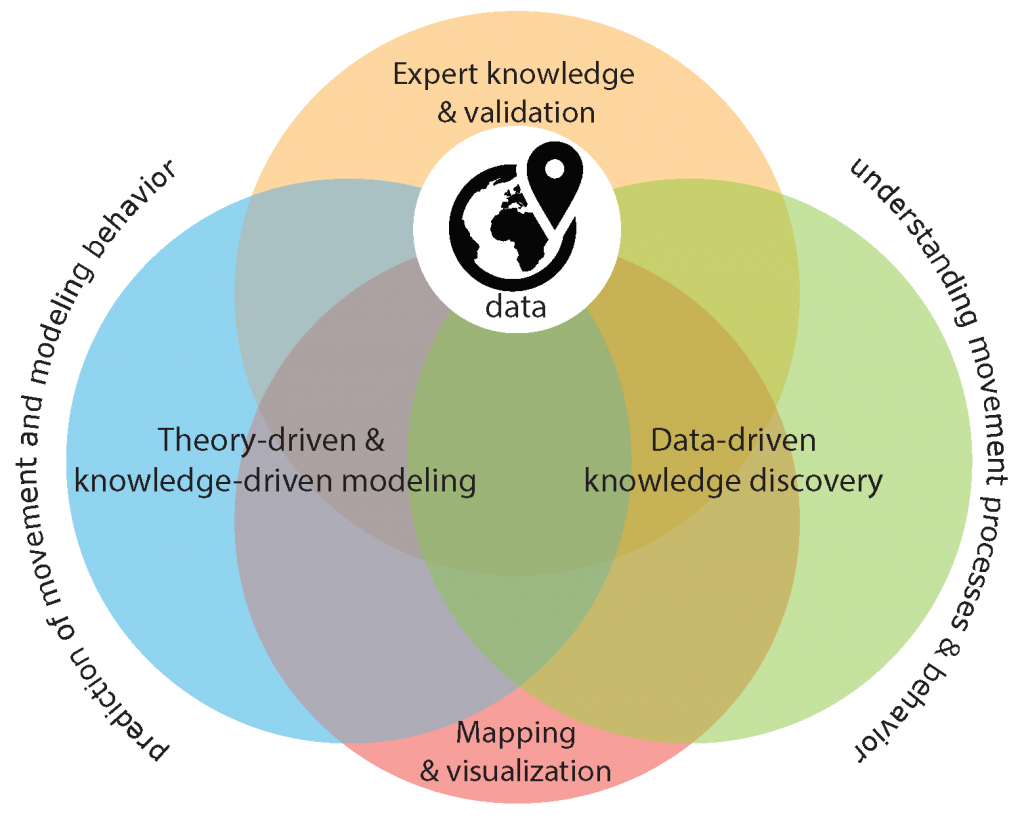
We leverage domain knowledge through interdisciplinary collaborations. We develop cutting-edge data-driven analytics, theory-driven computational models, and visualization techniques to analyze raw movement observations for meaningful knowledge discovery and predictions of movement. Below are some of the ongoing projects conducted by our team at MOVE@UCSB.
Understanding Human Environment Interaction through Movement
Our research focuses on developing quantitative geographic methodologies to explore the complex interactions between individuals and their environment across various spatial and temporal scales. We harness the power of large-scale, high-resolution mobile phone location data to advance our understanding and modeling of how environmental disruptions (e.g. wildfires and other natural hazards) impact daily movement patterns. Other projects investigate access to natural spaces and its subsequent effects on health and well-being. Through these innovative approaches, we aim to uncover valuable insights into human-environment relationships, ultimately contributing to more informed decision-making in urban planning, public health, and environmental management.